Algorithms for Data Science
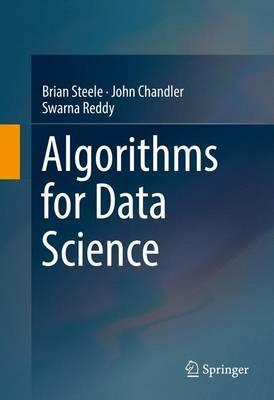
Algorithms for Data Science
This book has three parts:(a) Data Reduction: Begins with the concepts of data reduction, data maps, and information extraction. The second chapter introduces associative statistics, the mathematical foundation of scalable algorithms and distributed computing. Practical aspects of distributed computing is the subject of the Hadoop and MapReduce chapter.(b) Extracting Information from Data: Linear regression and data visualization are the principal topics of Part II. The authors dedicate a chapter to the critical domain of Healthcare Analytics for an extended example of practical data analytics. The algorithms and analytics will be of much interest to practitioners interested in utilizing the large and unwieldly data sets of the Centers for Disease Control and Prevention's Behavioral Risk Factor Surveillance System.(c) Predictive Analytics Two foundational and widely used algorithms, k-nearest neighbors and naive Bayes, are developed in detail. A chapter is dedicated to forecasting. The last chapter focuses on streaming data and uses publicly accessible data streams originating from the Twitter API and the NASDAQ stock market in the tutorials.
This book is intended for a one- or two-semester course in data analytics for upper-division undergraduate and graduate students in mathematics, statistics, and computer science. The prerequisites are kept low, and students with one or two courses in probability or statistics, an exposure to vectors and matrices, and a programming course will have no difficulty. The core material of every chapter is accessible to all with these prerequisites. The chapters often expand at the close with innovations of interest t
PRP: 652.72 Lei
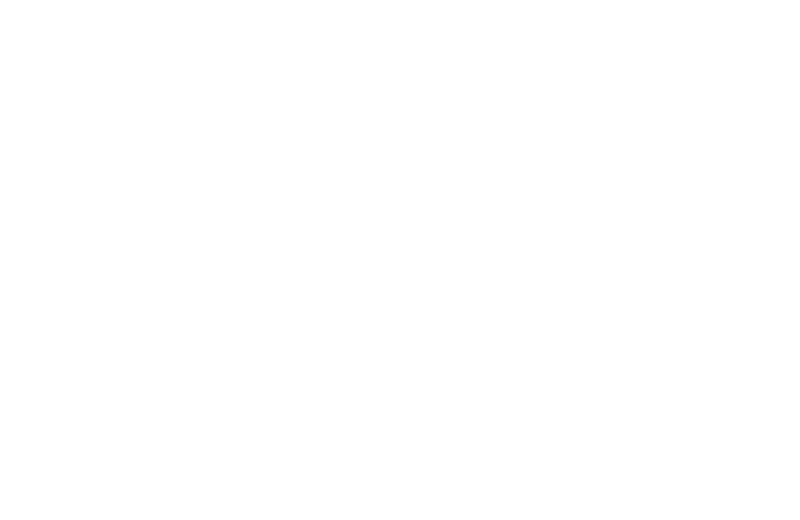
Acesta este Pretul Recomandat de Producator. Pretul de vanzare al produsului este afisat mai jos.
554.81Lei
554.81Lei
652.72 LeiLivrare in 2-4 saptamani
Descrierea produsului
This book has three parts:(a) Data Reduction: Begins with the concepts of data reduction, data maps, and information extraction. The second chapter introduces associative statistics, the mathematical foundation of scalable algorithms and distributed computing. Practical aspects of distributed computing is the subject of the Hadoop and MapReduce chapter.(b) Extracting Information from Data: Linear regression and data visualization are the principal topics of Part II. The authors dedicate a chapter to the critical domain of Healthcare Analytics for an extended example of practical data analytics. The algorithms and analytics will be of much interest to practitioners interested in utilizing the large and unwieldly data sets of the Centers for Disease Control and Prevention's Behavioral Risk Factor Surveillance System.(c) Predictive Analytics Two foundational and widely used algorithms, k-nearest neighbors and naive Bayes, are developed in detail. A chapter is dedicated to forecasting. The last chapter focuses on streaming data and uses publicly accessible data streams originating from the Twitter API and the NASDAQ stock market in the tutorials.
This book is intended for a one- or two-semester course in data analytics for upper-division undergraduate and graduate students in mathematics, statistics, and computer science. The prerequisites are kept low, and students with one or two courses in probability or statistics, an exposure to vectors and matrices, and a programming course will have no difficulty. The core material of every chapter is accessible to all with these prerequisites. The chapters often expand at the close with innovations of interest t
Detaliile produsului