Introduction to Graph Neural Networks
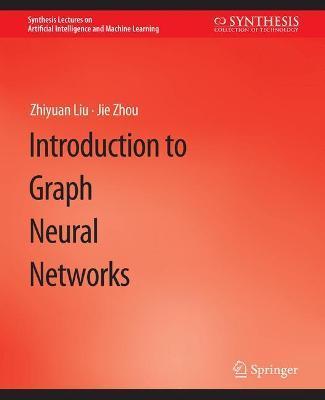
Introduction to Graph Neural Networks
Graphs are useful data structures in complex real-life applications such as modeling physical systems, learning molecular fingerprints, controlling traffic networks, and recommending friends in social networks. However, these tasks require dealing with non-Euclidean graph data that contains rich relational information between elements and cannot be well handled by traditional deep learning models (e.g., convolutional neural networks (CNNs) or recurrent neural networks (RNNs)). Nodes in graphs usually contain useful feature information that cannot be well addressed in most unsupervised representation learning methods (e.g., network embedding methods). Graph neural networks (GNNs) are proposed to combine the feature information and the graph structure to learn better representations on graphs via feature propagation and aggregation. Due to its convincing performance and high interpretability, GNN has recently become a widely applied graph analysis tool.
This book provides a comprehensive introduction to the basic concepts, models, and applications of graph neural networks. It starts with the introduction of the vanilla GNN model. Then several variants of the vanilla model are introduced such as graph convolutional networks, graph recurrent networks, graph attention networks, graph residual networks, and several general frameworks. Variants for different graph types and advanced training methods are also included. As for the applications of GNNs, the book categorizes them into structural, non-structural, and other scenarios, and then it introduces several typical models on solving these tasks. Finally, the closing chapters provide GNN open resources and the outlook of several future directions.PRP: 604.41 Lei
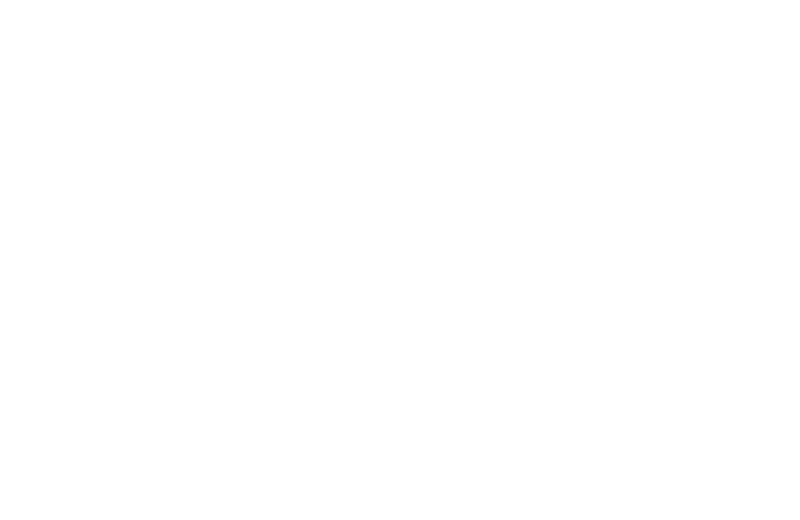
Acesta este Pretul Recomandat de Producator. Pretul de vanzare al produsului este afisat mai jos.
543.97Lei
543.97Lei
604.41 LeiLivrare in 2-4 saptamani
Descrierea produsului
Graphs are useful data structures in complex real-life applications such as modeling physical systems, learning molecular fingerprints, controlling traffic networks, and recommending friends in social networks. However, these tasks require dealing with non-Euclidean graph data that contains rich relational information between elements and cannot be well handled by traditional deep learning models (e.g., convolutional neural networks (CNNs) or recurrent neural networks (RNNs)). Nodes in graphs usually contain useful feature information that cannot be well addressed in most unsupervised representation learning methods (e.g., network embedding methods). Graph neural networks (GNNs) are proposed to combine the feature information and the graph structure to learn better representations on graphs via feature propagation and aggregation. Due to its convincing performance and high interpretability, GNN has recently become a widely applied graph analysis tool.
This book provides a comprehensive introduction to the basic concepts, models, and applications of graph neural networks. It starts with the introduction of the vanilla GNN model. Then several variants of the vanilla model are introduced such as graph convolutional networks, graph recurrent networks, graph attention networks, graph residual networks, and several general frameworks. Variants for different graph types and advanced training methods are also included. As for the applications of GNNs, the book categorizes them into structural, non-structural, and other scenarios, and then it introduces several typical models on solving these tasks. Finally, the closing chapters provide GNN open resources and the outlook of several future directions.Detaliile produsului