Mathematical Foundations of Infinite-Dimensional Statistical Models
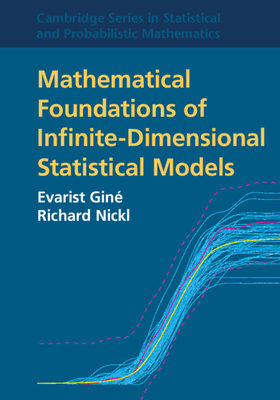
Mathematical Foundations of Infinite-Dimensional Statistical Models
In nonparametric and high-dimensional statistical models, the classical Gauss-Fisher-Le Cam theory of the optimality of maximum likelihood estimators and Bayesian posterior inference does not apply, and new foundations and ideas have been developed in the past several decades. This book gives a coherent account of the statistical theory in infinite-dimensional parameter spaces. The mathematical foundations include self-contained 'mini-courses' on the theory of Gaussian and empirical processes, approximation and wavelet theory, and the basic theory of function spaces. The theory of statistical inference in such models - hypothesis testing, estimation and confidence sets - is presented within the minimax paradigm of decision theory. This includes the basic theory of convolution kernel and projection estimation, but also Bayesian nonparametrics and nonparametric maximum likelihood estimation. In a final chapter the theory of adaptive inference in nonparametric models is developed, including Lepski's method, wavelet thresholding, and adaptive inference for self-similar functions. Winner of the 2017 PROSE Award for Mathematics.
PRP: 465.43 Lei
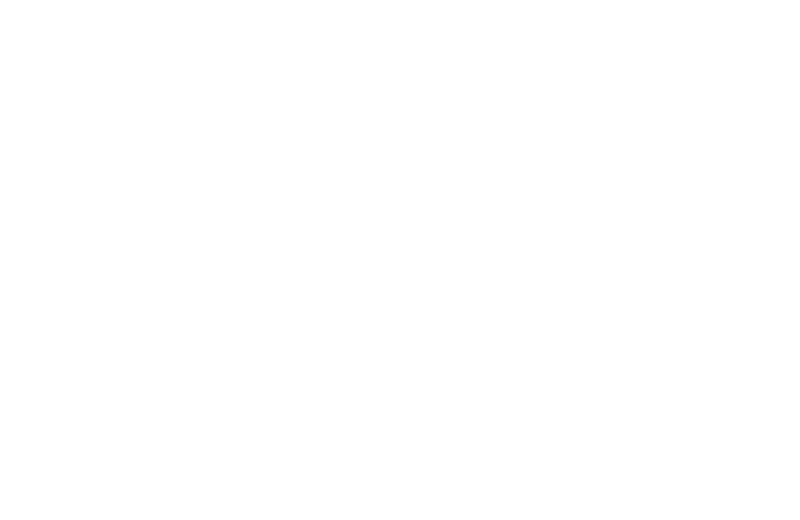
Acesta este Pretul Recomandat de Producator. Pretul de vanzare al produsului este afisat mai jos.
418.89Lei
418.89Lei
465.43 LeiLivrare in 2-4 saptamani
Descrierea produsului
In nonparametric and high-dimensional statistical models, the classical Gauss-Fisher-Le Cam theory of the optimality of maximum likelihood estimators and Bayesian posterior inference does not apply, and new foundations and ideas have been developed in the past several decades. This book gives a coherent account of the statistical theory in infinite-dimensional parameter spaces. The mathematical foundations include self-contained 'mini-courses' on the theory of Gaussian and empirical processes, approximation and wavelet theory, and the basic theory of function spaces. The theory of statistical inference in such models - hypothesis testing, estimation and confidence sets - is presented within the minimax paradigm of decision theory. This includes the basic theory of convolution kernel and projection estimation, but also Bayesian nonparametrics and nonparametric maximum likelihood estimation. In a final chapter the theory of adaptive inference in nonparametric models is developed, including Lepski's method, wavelet thresholding, and adaptive inference for self-similar functions. Winner of the 2017 PROSE Award for Mathematics.
Detaliile produsului